Issue #127 – On the Sparsity of Neural MT Models
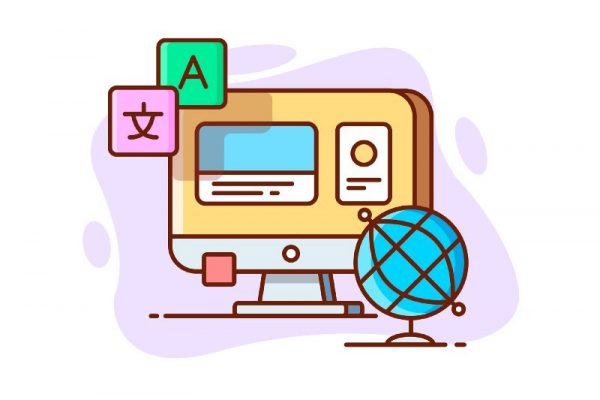
22 Apr21
Issue #127 – On the Sparsity of Neural MT Models
Author: Dr. Jingyi Han, Machine Translation Scientist @ Iconic
Introduction
Looking at the evolution of Neural Machine Translation (NMT), from a simple feed-forward approach to the recent state of the art Transformer architecture, models are getting more and more complicated by involving a large number of parameters to fit a massive data well. As a consequence, over-parameterization is a common problem suffered by NMT models, and it is certainly a waste of computational resources. Some recent research (e.g. See et al., 2016 and Lan et al., 2020) demonstrates that a significant part of the parameters can be pruned without sacrificing translation performance. In this post, we take a look at