Self-Explaining Structures Improve NLP Models
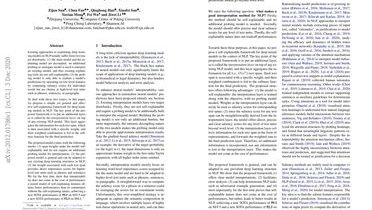
Existing approaches to explaining deep learning models in NLP usually suffer from two major drawbacks: (1) the main model and the explaining model are decoupled: an additional probing or surrogate model is used to interpret an existing model, and thus existing explaining tools are not self-explainable; (2) the probing model is only able to explain a model’s predictions by operating on low-level features by computing saliency scores for individual words but are clumsy at high-level text units such as phrases, sentences, or paragraphs. To deal with these two issues, in this paper, we propose a simple yet general