Learning Semantic-aware Normalization for Generative Adversarial Networks
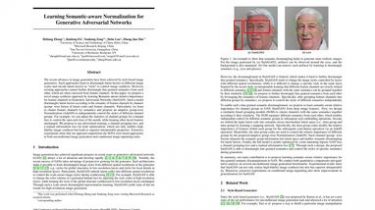
The recent advances in image generation have been achieved by style-based image generators. Such approaches learn to disentangle latent factors in different image scales and encode latent factors as “style” to control image synthesis...
However, existing approaches cannot further disentangle fine-grained semantics from each other, which are often conveyed from feature channels. In this paper, we propose a novel image synthesis approach by learning Semantic-aware relative importance for feature channels in Generative Adversarial Networks (SariGAN). Such a model disentangles latent factors according to the semantic of feature channels by channel-/group- wise fusion of latent codes and