Learnable Boundary Guided Adversarial Training
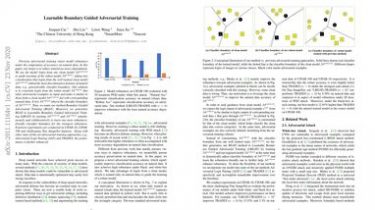
Previous adversarial training raises model robustness under the compromise of accuracy on natural data. In this paper, our target is to reduce natural accuracy degradation...
We use the model logits from one clean model $mathcal{M}^{natural}$ to guide learning of the robust model $mathcal{M}^{robust}$, taking into consideration that logits from the well trained clean model $mathcal{M}^{natural}$ embed the most discriminative features of natural data, {it e.g.}, generalizable classifier boundary. Our solution is to constrain logits from the robust model $mathcal{M}^{robust}$ that takes adversarial examples as input and make it similar to those from a clean model $mathcal{M}^{natural}$