Conflicting Bundles: Adapting Architectures Towards the Improved Training of Deep Neural Networks
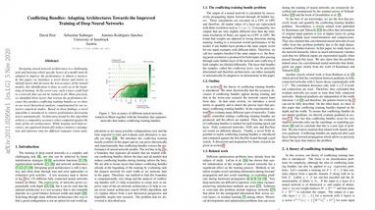
Designing neural network architectures is a challenging task and knowing which specific layers of a model must be adapted to improve the performance is almost a mystery. In this paper, we introduce a novel theory and metric to identify layers that decrease the test accuracy of the trained models, this identification is done as early as at the beginning of training...
In the worst-case, such a layer could lead to a network that can not be trained at all. More precisely, we identified those layers that worsen the performance because they produce conflicting training bundles as