Intriguing Properties of Contrastive Losses
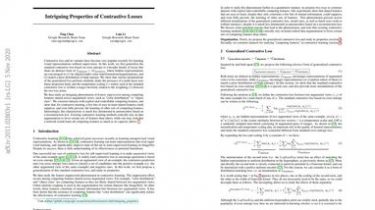
Contrastive loss and its variants have become very popular recently for learning visual representations without supervision. In this work, we first generalize the standard contrastive loss based on cross entropy to a broader family of losses that share an abstract form of $mathcal{L}_{text{alignment}} + lambda mathcal{L}_{text{distribution}}$, where hidden representations are encouraged to (1) be aligned under some transformations/augmentations, and (2) match a prior distribution of high entropy...
We show that various instantiations of the generalized loss perform similarly under the presence of a multi-layer non-linear projection head, and the temperature scaling ($tau$) widely used in the