Exploring DeshuffleGANs in Self-Supervised Generative Adversarial Networks
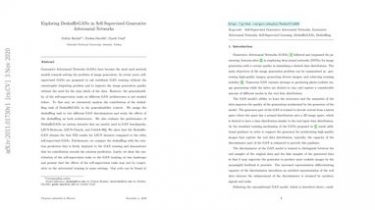
Generative Adversarial Networks (GANs) have become the most used network models towards solving the problem of image generation. In recent years, self-supervised GANs are proposed to aid stabilized GAN training without the catastrophic forgetting problem and to improve the image generation quality without the need for the class labels of the data...
However, the generalizability of the self-supervision tasks on different GAN architectures is not studied before. To that end, we extensively analyze the contribution of the deshuffling task of DeshuffleGANs in the generalizability context. We assign the deshuffling task to two different GAN discriminators and