Attentive Clustering Processes
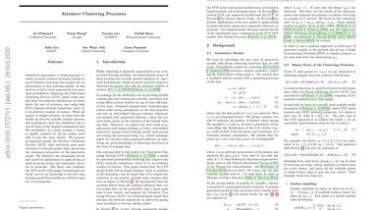
Amortized approaches to clustering have recently received renewed attention thanks to novel objective functions that exploit the expressiveness of deep learning models. In this work we revisit a recent proposal for fast amortized probabilistic clustering, the Clusterwise Clustering Process (CCP), which yields samples from the posterior distribution of cluster labels for sets of arbitrary size using only O(K) forward network evaluations, where K is an arbitrary number of clusters...
While adequate in simple datasets, we show that the model can severely underfit complex datasets, and hypothesize that this limitation can be traced back to the implicit