Displacement-Invariant Matching Cost Learning for Accurate Optical Flow Estimation
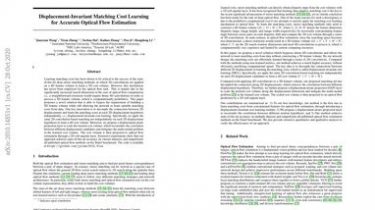
Learning matching costs has been shown to be critical to the success of the state-of-the-art deep stereo matching methods, in which 3D convolutions are applied on a 4D feature volume to learn a 3D cost volume. However, this mechanism has never been employed for the optical flow task...
This is mainly due to the significantly increased search dimension in the case of optical flow computation, ie, a straightforward extension would require dense 4D convolutions in order to process a 5D feature volume, which is computationally prohibitive. This paper proposes a novel solution that is able to