4 Automatic Outlier Detection Algorithms in Python
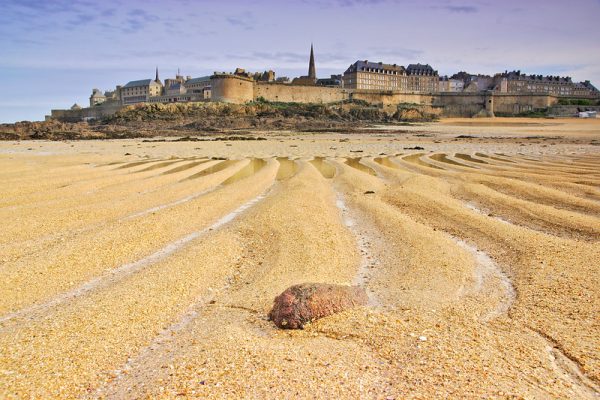
Last Updated on August 17, 2020
The presence of outliers in a classification or regression dataset can result in a poor fit and lower predictive modeling performance.
Identifying and removing outliers is challenging with simple statistical methods for most machine learning datasets given the large number of input variables. Instead, automatic outlier detection methods can be used in the modeling pipeline and compared, just like other data preparation transforms that may be applied to the dataset.
In this tutorial, you will discover how to use automatic outlier detection and removal to improve machine learning predictive modeling performance.
After completing this tutorial, you will know:
- Automatic outlier detection models provide an alternative to statistical techniques with a larger number of input variables with complex and unknown inter-relationships.
- How to correctly apply automatic outlier detection and removal to the training dataset only to avoid data leakage.
- How to evaluate and compare predictive modeling pipelines with outliers removed from the training dataset.
Kick-start your project with my new book Data Preparation for Machine Learning, including step-by-step tutorials and the Python source code files for all examples.
Let’s get started.
