6 Dimensionality Reduction Algorithms With Python
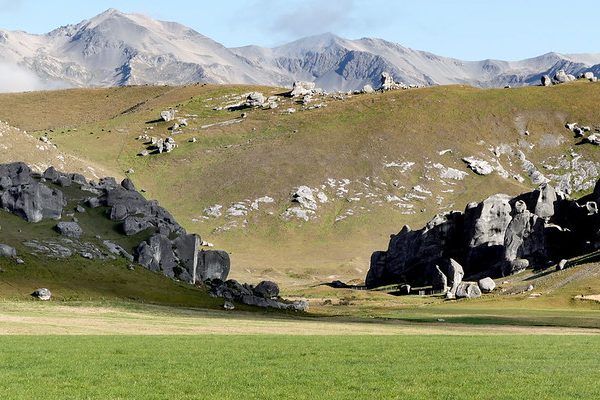
Last Updated on August 17, 2020
Dimensionality reduction is an unsupervised learning technique.
Nevertheless, it can be used as a data transform pre-processing step for machine learning algorithms on classification and regression predictive modeling datasets with supervised learning algorithms.
There are many dimensionality reduction algorithms to choose from and no single best algorithm for all cases. Instead, it is a good idea to explore a range of dimensionality reduction algorithms and different configurations for each algorithm.
In this tutorial, you will discover how to fit and evaluate top dimensionality reduction algorithms in Python.
After completing this tutorial, you will know:
- Dimensionality reduction seeks a lower-dimensional representation of numerical input data that preserves the salient relationships in the data.
- There are many different dimensionality reduction algorithms and no single best method for all datasets.
- How to implement, fit, and evaluate top dimensionality reduction in Python with the scikit-learn machine learning library.
Kick-start your project with my new book Data Preparation for Machine Learning, including step-by-step tutorials and the Python source code files for all examples.
Let’s get started.
