5 Useful Loss Functions
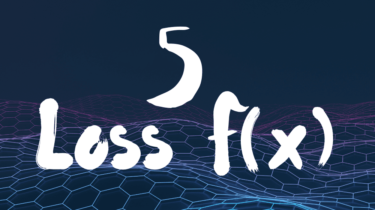
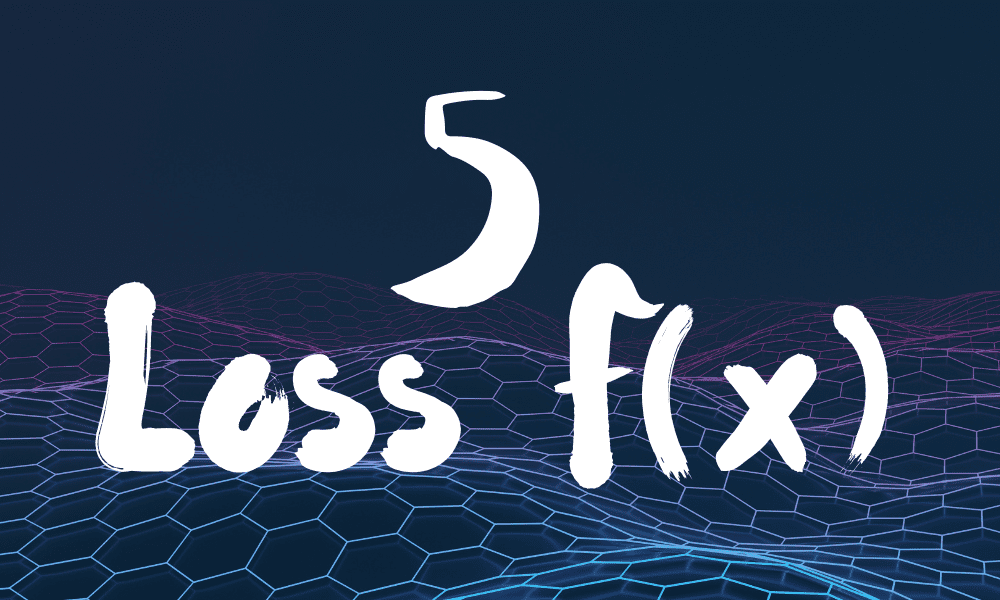
Image by Author
A loss function in machine learning is a mathematical formula that calculates the difference between the predicted output and the actual output of the model. The loss function is then used to slightly change the model weights and then check whether it has improved the model’s performance. The goal of machine learning algorithms is to minimize the loss function in order to make accurate predictions.
In this blog, we will learn about the 5 most commonly used loss functions for classification and regression machine learning algorithms.
1. Binary Cross-Entropy Loss
Binary cross-entropy loss, or Log loss, is a commonly used loss function