5 Effective Ways to Handle Imbalanced Data in Machine Learning
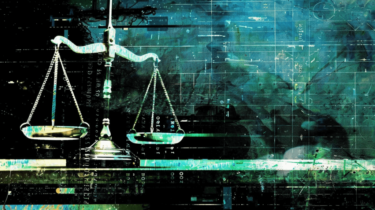
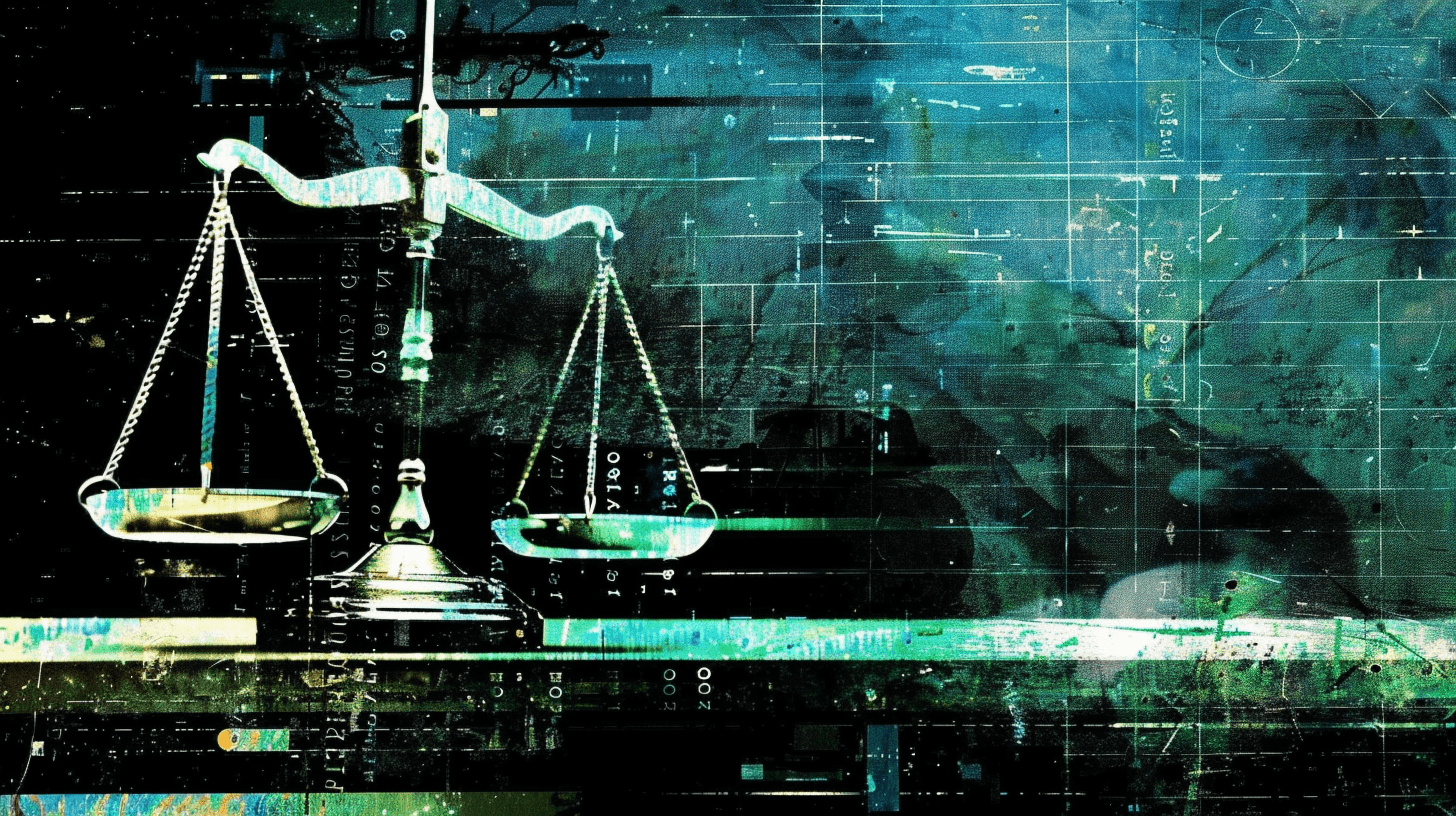
Image by Author
Introduction
Here’s a something that new machine learning practitioners figure out almost immediately: not all datasets are created equal.
It may now seem obvious to you, but had you considered this before undertaking machine learning projects on a real world dataset? As an example of a single class vastly outnumbering the rest, take for instance some rare disease, which only 1% of the population has. Would a predictive model that only ever predicts “no disease” still be thought of as beneficial even if it is 99% correct? Of course not.
In machine learning, imbalanced datasets can be obstacles